Self-checking AI: A trustworthy knowledge assistant for the energy sector with 90% user satisfaction
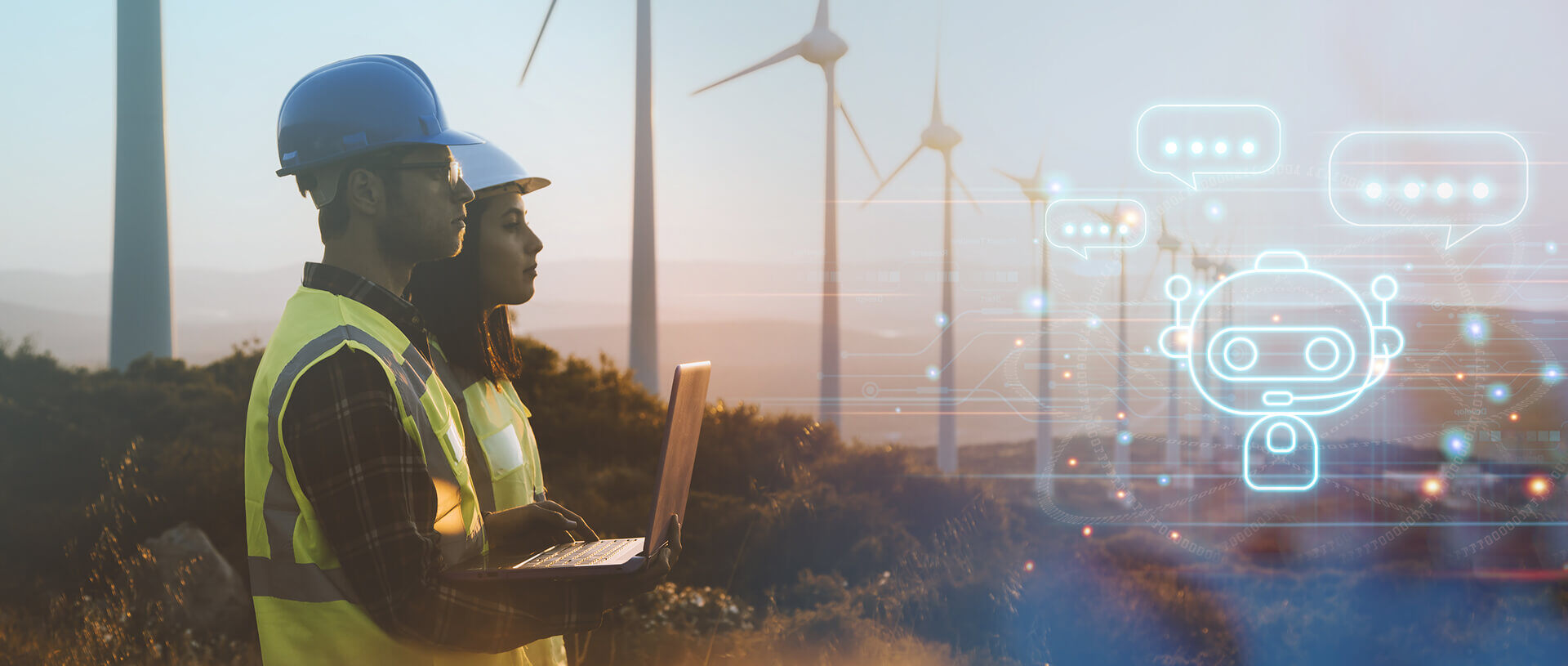
Self-Checking AI Chatbot: A Game Changer for Customer Support
This is a hidden post summary. It displays in the editor only.*
Our latest case study showcases how SUNZINET helped a leading energy provider implement an AI-powered chatbot that centralizes knowledge, enhances efficiency, and ensures accuracy with a self-checking AI model. The result?
- 90%+ user satisfaction among employees
- <1% misleading answers, ensuring high trust
- Faster customer service & streamlined onboarding
- AI-powered self-evaluation to minimize errors
Read the full success story & discover how an AI solution transforms knowledge management in customer service!
The Project
Overcoming information silos
Our customer, a major player in the energy sector, manages a vast, international customer base. Since energy and gas utilities are complicated services, there is a lot of pressure on customer-facing teams, including call centres, sales representatives, contract management, and claims departments. These teams are responsible for handling thousands of inquiries, contracts, and claims every month, all while lacking a centralized, single source of truth.
All the employees especially the Service teams, relied on scattered, inconsistent sources to find the information they need, leading to:
❌ Longer response times
❌ Increased workload
❌ Potential revenue loss
So, the customer contacted our AI Experts, to resolve these challenges.
-
KPIs
User satisfaction rate > 90%
-
Project Timeline
March-November 2024
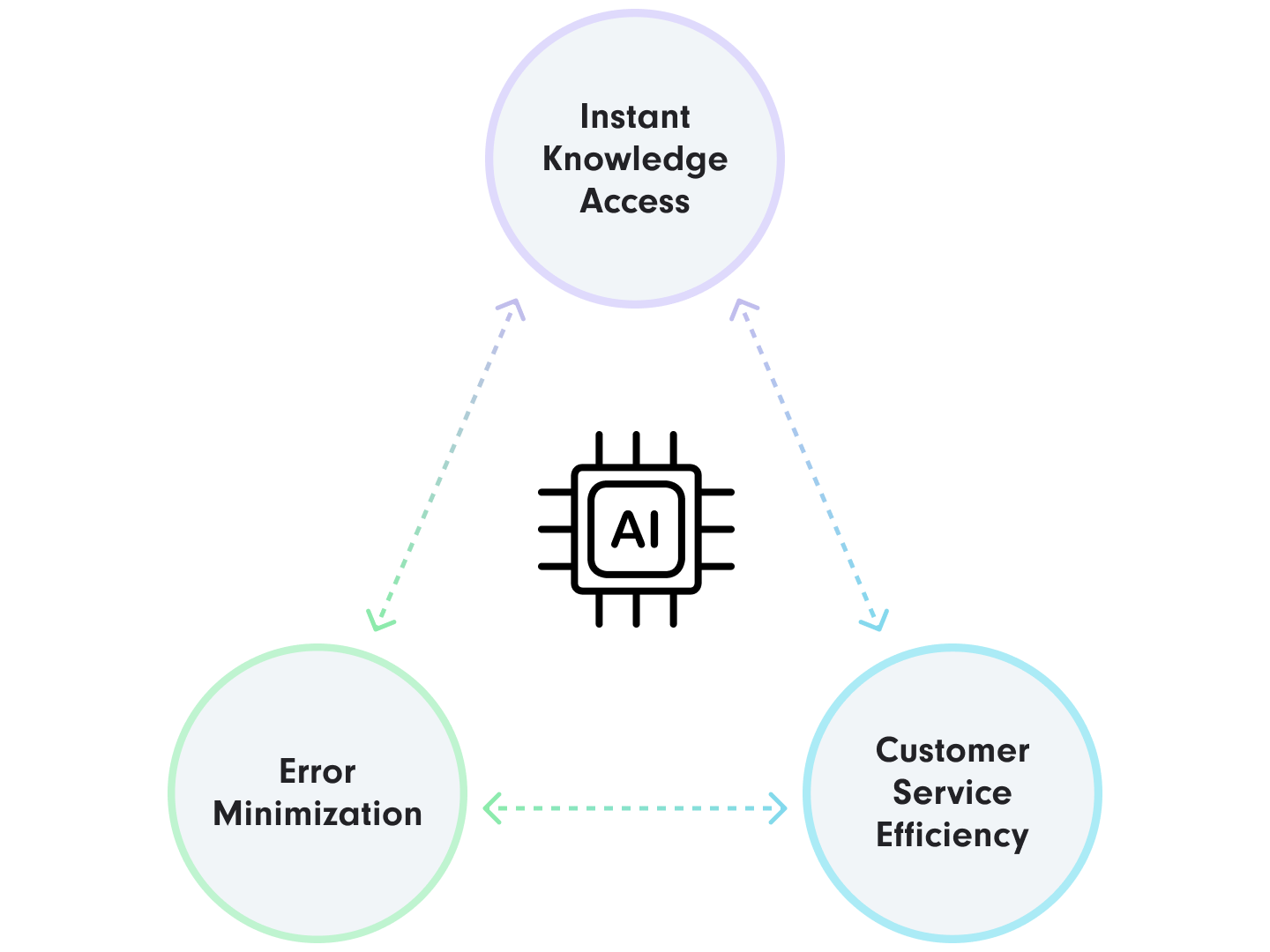
The Goal of the Project
Smarter knowledge management with AI
The project aimed to create a Proof of Concept (PoC) version of an
AI assistant that would centralize knowledge and make it readily accessible.
Implementing the full version of this solution would increase productivity, enhance decision-making, and improve the overall customer experience, all while ensuring accuracy and security in information management.
The Key goals included:
- Provide instant and reliable access to critical knowledge – reducing reliance on scattered documents and colleagues for information retrieval.
- Improve customer service efficiency – enabling first-line teams to resolve more inquiries independently, reducing escalations and handling times.
- Minimize errors in contract verification and documentation – reducing rejected contracts and revenue loss due to incorrect paperwork.
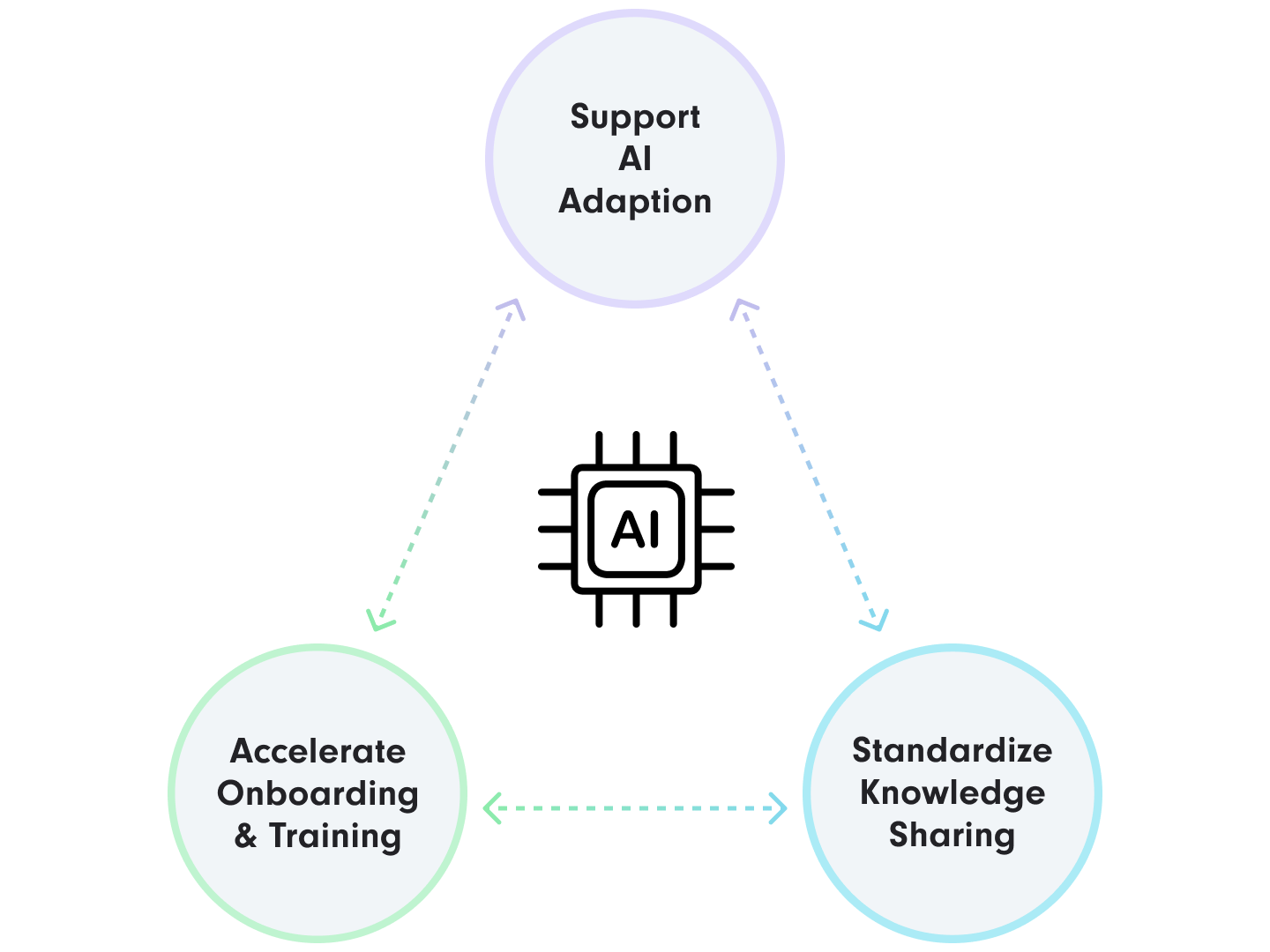
- Standardize knowledge sharing across departments – replacing informal processes with a structured, AI-driven knowledge base integrated into existing systems.
- Accelerate onboarding and training – ensuring new employees can quickly access accurate information and minimize the need for 1:1 coaching
- Support AI adoption and future scalability – lay the foundation for expanding AI support to both employees and customers over time.
Key inefficiencies that our AI knowledge Management Solution Solved
Knowledge silos
Critical information is stored across USYS (ERP/CRM system), SharePoint, MS Teams, and Outlook emails, making searchability difficult.
Reliance on individual expertise
Employees frequently ask colleagues for answers instead of retrieving information from a structured database. This is particularly inefficient in remote work setups.
High onboarding volume
With an annual turnover rate of 20-35% in the call centre, maintaining knowledge consistency and training new employees efficiently requires significant resources.
Documentation inconsistencies
Different teams maintain knowledge separately, resulting in errors, delays, and miscommunication between departments.
Strategy & Implementation
To ensure a successful deployment of the AI-powered knowledge assistant, SUNZINET followed a structured approach that combined a Proof of Concept (PoC), iterative testing, and rigorous evaluation metrics.
-
SUNZINET and the client collaboratively developed a PoC within a selected department to refine the AI knowledge assistant before scaling.
- Building the knowledge base: A test database with 100 curated Q&A pairs was structured into multiple segments: B2B vs. B2C; Frontline vs. Second-line teams (simple vs. detailed documents); Department-specific repositories.
- Defining success metrics: Accuracy, usability, and efficiency thresholds were set with the client.
- Cloud setup: The AI assistant was deployed in Azure OpenAI Service, ensuring security, compliance, and scalability.
-
- Selecting the AI model: Various Language Models were evaluated for accuracy, efficiency, and cost-effectiveness.
- Designing the query process: The AI assistant was optimized using a chain of prompts to ensure it interprets, processes, and retrieves relevant knowledge efficiently.
- Prompt engineering & response formatting: Commands were optimized to generate structured, easy-to-read responses tailored to real-world usage.
-
- Document structuring: Large documents were split into searchable segments.
- Metadata enhancement: Documents were enriched with metadata for better indexing and retrieval.
- Database configuration: A vector database was implemented to enable semantic search capabilities, improving response relevance.
- Optimized retrieval: AI pulls the most relevant information efficiently.
-
- Test script execution: The AI assistant was tested against a scripted set of real-world questions.
- Dual-layer evaluation:
- AI self-assessment on response accuracy.
- Human review of AI-generated answers.
-
- User interface: Designed for easy employee interaction.
- Feedback loop: Sales and service teams tested AI, refining accuracy.
- Final report: Summarized AI performance, key learnings, and next steps for scaling.
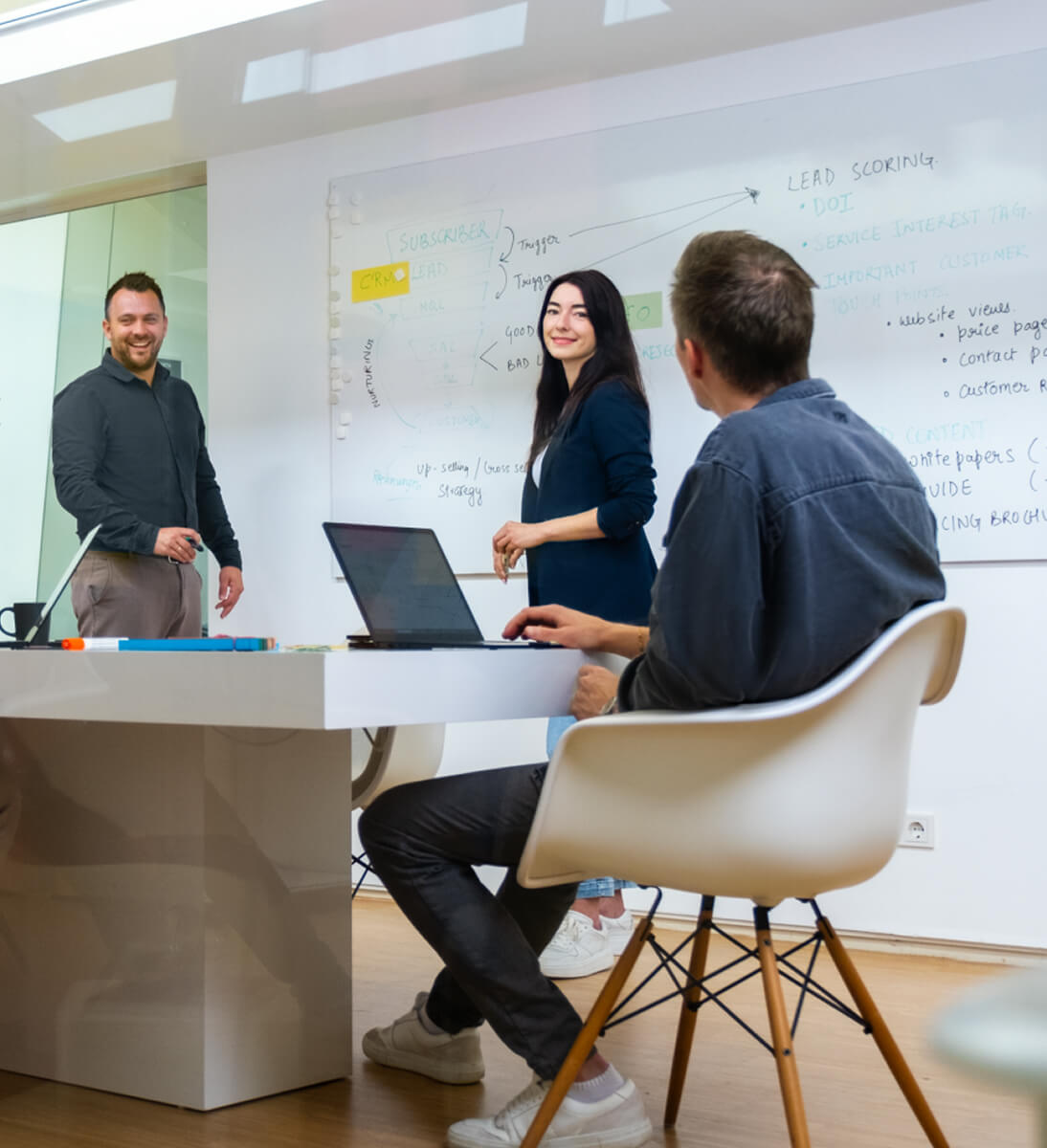
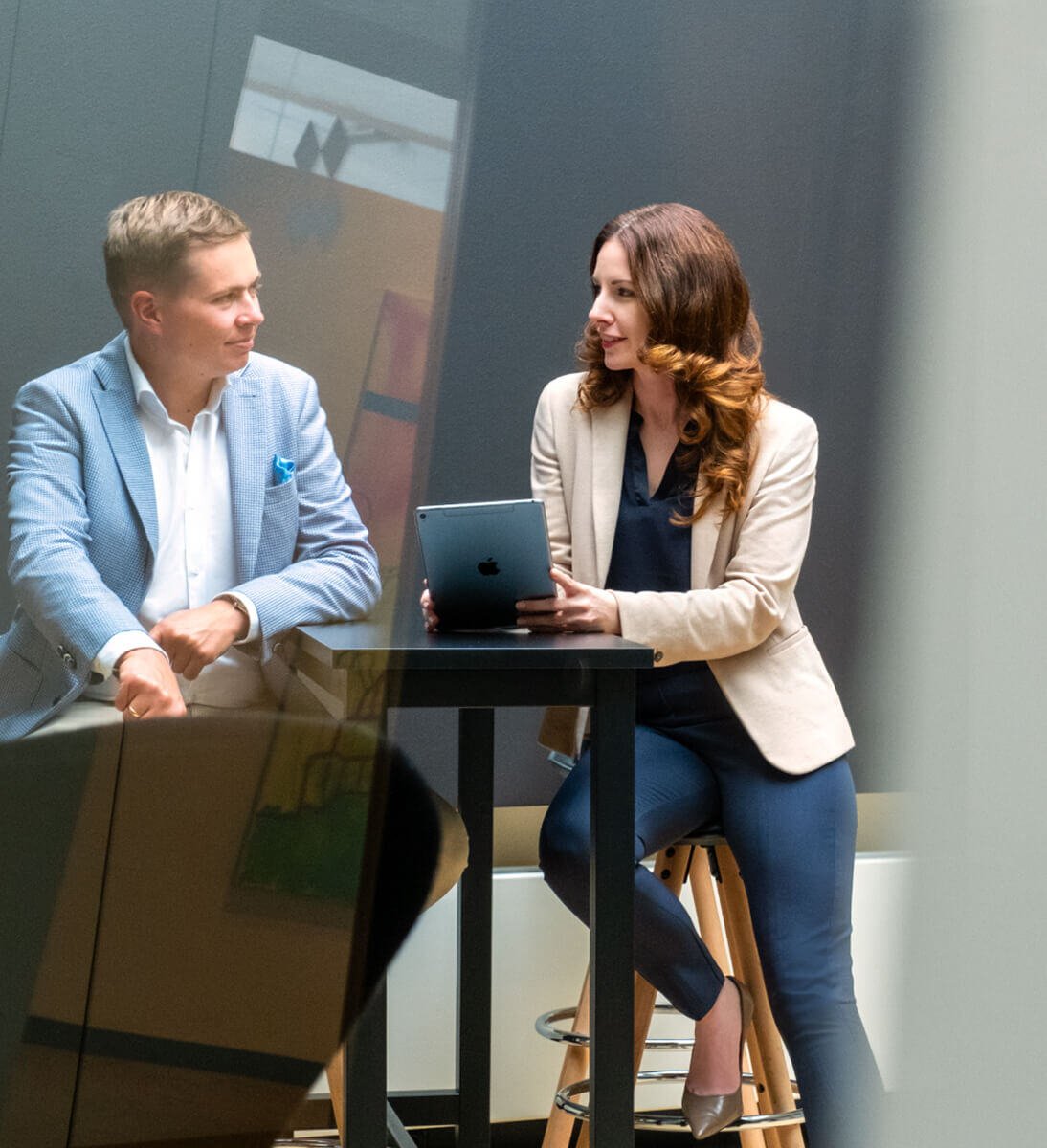
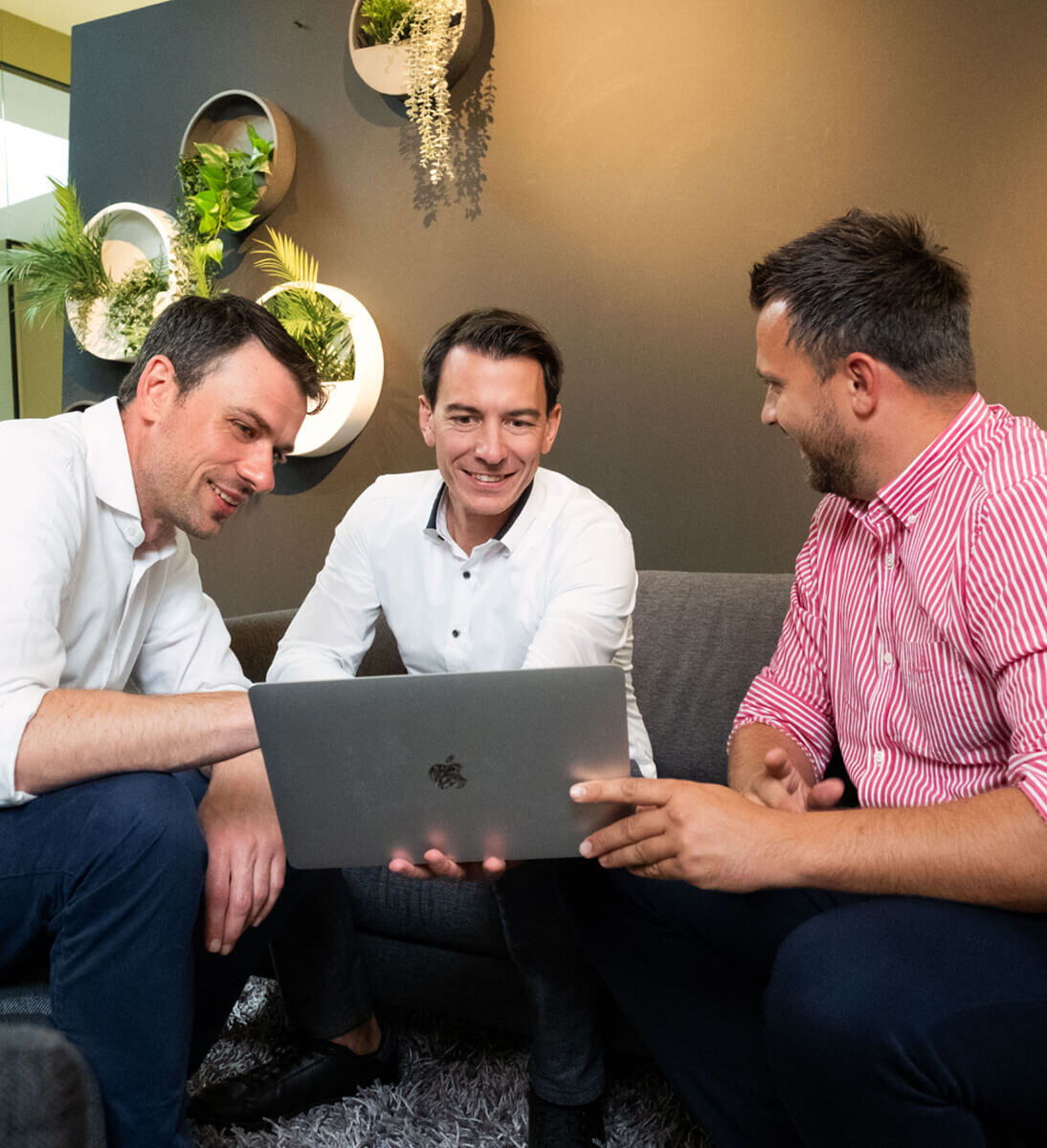
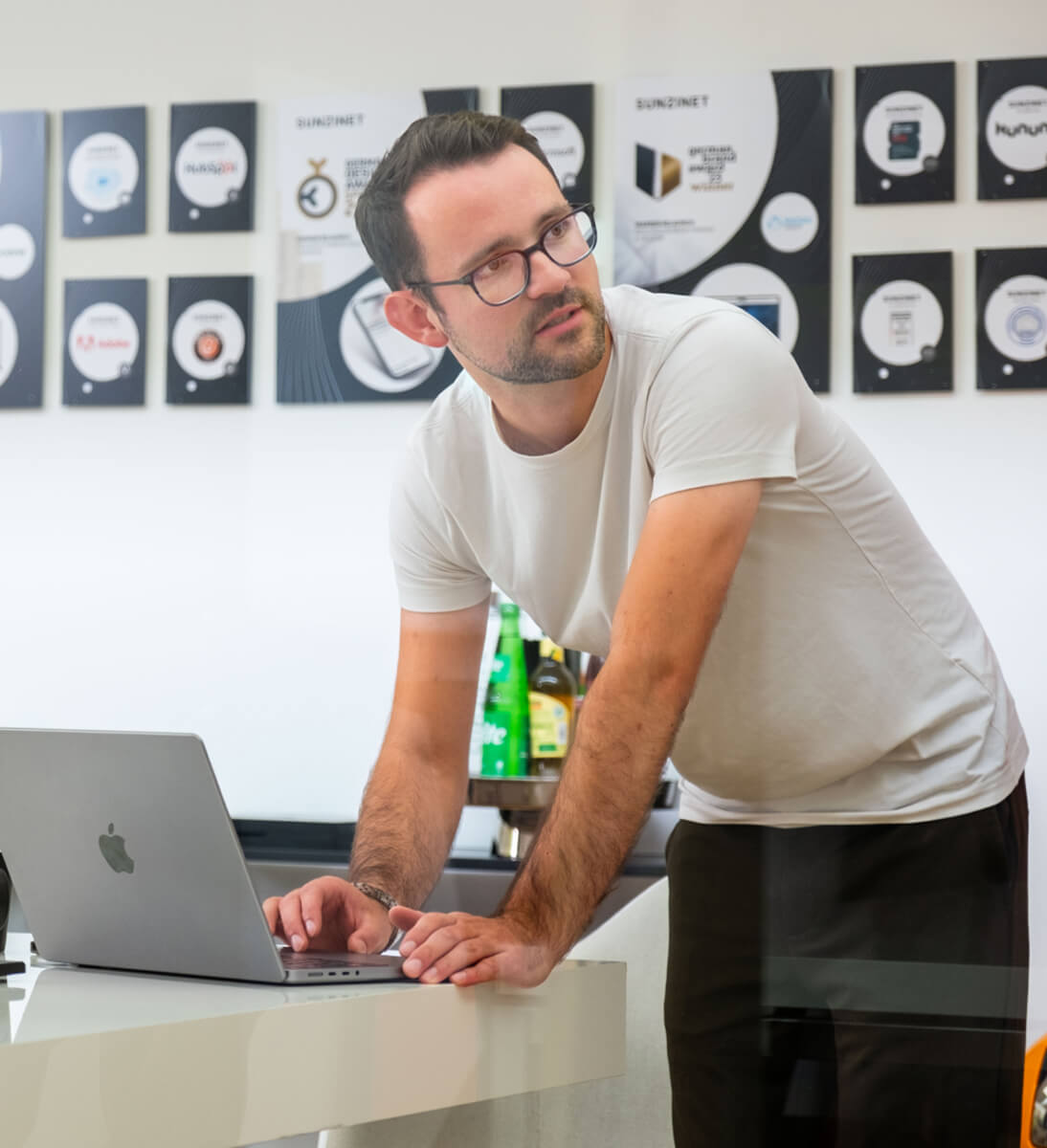
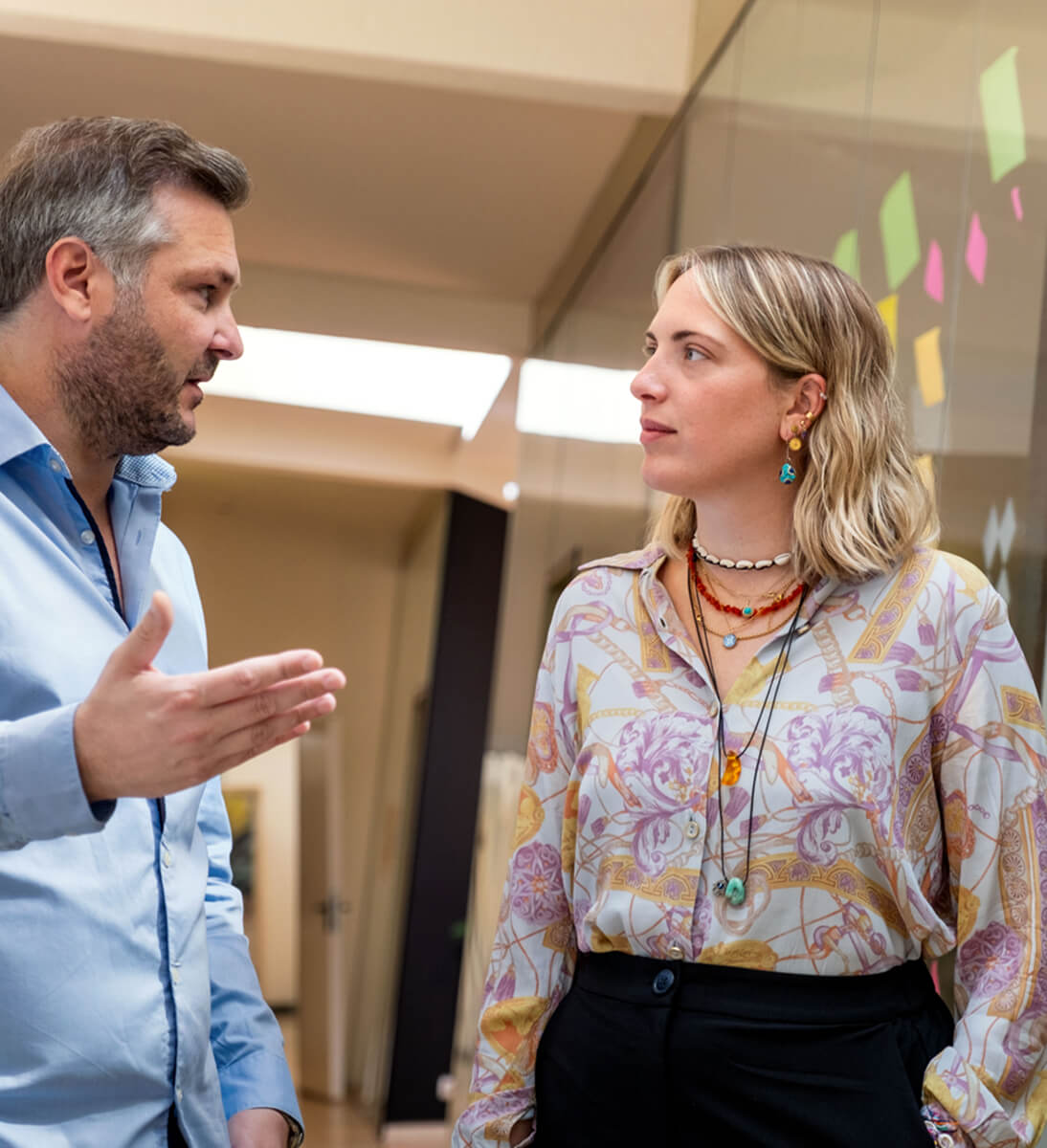
Results & Impact
AI knowledge Management Chatbot - A Game changer for Service Teams
We deployed the PoC of the AI assistant successfully with the following functionalities:
- An AI-powered chatbot that intelligently searches a knowledge base and delivers accurate answers. It was tested with predefined questions to ensure reliability before deployment.
- Structured knowledge base managed by SUNZINET, ensuring it contains relevant, curated information for accurate responses. The data was preloaded before deployment, so the chatbot could provide instant answers without needing live data input.
- User authentication system that controls who can access the AI assistant. Access permissions are managed to ensure that different users (e.g., support agents, managers) can only view or modify information based on their roles.
Value for the Customer
Key Performance Metrics
- User satisfaction rate: Over 90% of testers said they like to use the tool in their daily work.
- Incorrect answer rate: ~8%, with almost all incorrect responses flagged by the AI’s self-check.
- Misleading answer rate: <1%, ensuring high reliability.
Project Highlight: Trust & Accuracy
The AI assistant’s ability to self-evaluate its responses sets this project apart. By verifying its own accuracy, and prompting for clarifications when uncertain, the tool increased user confidence, making it a highly trusted resource for employees.
This high-quality AI response system, combined with structured knowledge access, resulted in a successful PoC with strong adoption potential for full-scale implementation.
This trailblazing project underscores the AI assistant’s potential to enhance service efficiency, streamline knowledge management, and drive smarter decision-making across the organization.